This document
This document is intended to be an open and evolving draft that reflects the current thinking around Open Urban Digital Twins. At certain points in time, a pdf will be created and circulated over various media. Anyone is warmly invited to review and contribute to the document. Urban Digital Twins are also referred to as Local Digital Twins. We proposed that most of what is discussed in this paper also applies to Local Digital Twins.
Open Urban Digital Twins
In this paper, we bundle and explain insights on the added value, architecture and design of Urban Digital Twins as cross-domain urban decision support systems. In doing so, we aim to facilitate a common understanding of the concept of Urban Digital Twins. Such an understanding is much needed, as Urban Digital Twins are currently defined and understood in different ways by different people. We hope this paper will help readers, especially from the public and private sector, to consolidate a common understanding of the topic.
In recent years, the idea to build Urban Digital Twins representing entire cities and regions has gained increasing attention. Yet, as cities consist of complex ecosystems, it is not straightforward to build such Urban Digital Twins. Questions arise about how Urban Digital Twins can exactly be used to support decision making processes within the local government and how this affects design principles and architecture. These are the overall questions which we aim to address in this paper, in addition to establishing a common understanding of what Urban Digital Twins are and what they can be used for.
Digital Twins in general
Since its inception around the turn of the century, the definition of a Digital Twin has been debated and challenged by various authors (e.g. Grieves and Vickers 2017, Alam and El Saddik 2017, Tao et al. 2018, Zheng et al. 2019). One of these definitions, which we will adopt in this paper, describes a Digital Twin as a “virtual representation of a physical entity with a bi-directional communication link” (adapted from Tao et al 2018). One of the key terms in this definition is the bi-directional communication link, which can be split-up into a communication link from the physical entity to the Digital Twin and a communication link from the Digital Twin back to the physical entity.
Communication link from the physical to the Digital Twin
The communication link from the physical to the Digital Twin refers to a constant use of several data collection techniques and devices (like sensors connected through Internet of Things technology) to collect and combine data about the physical entity. This data allows the virtual Digital Twin to constantly learn from and evolve along with its physical counterpart, mirroring its lifecycle. As a result, a Digital Twin can be used to get insights in the current state of the physical enitiy as well as predict future states of the physical entity through causal data models and simulation algorithms.
Communication link from the Digital to the physical twin
The Digital Twin may transform the physical entity by either automatically driving actuators connected through Internet-of-Things technology or by informing human decision makers who can make adaptations to the physical entity. The latter can be made through daily operational management or through long-term policy planning. In either case, the Digital Twin should be able to capture the changes made to the physical entity so that it can use those changes and their impact to update its data models. Thus a Digital Twin is not merely a detached virtual representation of a physical twin, but rather an evolving representation that constantly interacts with - and tests the impact of - novel configurations in the physical entity that it represents.
Local Digital Twins
With our improved understanding of Digital Twins in general, we can now better understand what is intended by a "Local" Digital Twin: it is a version where the physical entity is a city, a rural or a local entity and which is used to support decisions that pertain to this location. We will define them as: "Local Digital twins are dynamic, data driven, multi-dimensional digital replica of a physical rural, local or urban entity. They encompass potential or actual physical assets, processes, people, places, systems, devices and the natural environment. Their aim is to capture reality for supporting reactive and predictive decision making processes at relevant decision making levels in order to ensure minimum environmental impact, improved quality of life and enhanced performance of the local entity." Urban Digital Twins are just one type of urban decision support systems, next to information systems like Geographical Information Systems (GIS) and IoT data dashboards. Given these different types of already-existing systems, the question arises whether there is a need to create Urban Digital Twins and, more specifically, which distinguishing tasks such Digital Twins could fulfil. These are the kind of questions we have been discussing with multiple local urban decision makers through a number of workshops (with the cities of Bruges, Antwerp, Ghent, Pilzen, Athens and the Flemish region) from which emanated a list of possible use case descriptions. Each use case was formulated as a “how could we” question, for example “How could we quickly evaluate the water quality of a waterway to find out if it is safe to swim?”. The results of these insights were translated into a typology of the functional scope of an Urban Digital Twin:
- Decision support scope
- Design
- Operations
- Human decision making
- Automated decision making
- Intervention evaluation
- Decision support horizon
- Short
- Medium
- Long
- Decision support domain
- Air quality
- Mobility
- Sound
- Water
- Pandemics
- Tourism
- Urban planning
- End-users
- Policy makers
- Public servants
- Urban planner
- Emergency responder
- Citizen
Decision support scope
In terms of decision support, a first type of use cases are those that aim to make changes to the physical space of the city itself by redesigning it. These for example include the support of decisions on where to create infrastructure that would prevent certain areas of the city to suffer from floods due to heavy rain. These are typically use cases where the decisions that are supported are implemented over a longer period of time. A second type of decision-support use case occurs in the daily operations of the city, where decisions are made based on an up-to-date operational insight in the state of the city. An example would be to achieve a better understanding of the numbers of tourists at certain locations in the city, in order to take measures related to COVID-19. In such use cases, decisions can be taken and actuated by a human, or by a machine. In the latter case, an Urban Digital Twin could for example be used to build an up-to-date overview of the city, which serves to change the state of traffic lights. In such a use case, an algorithm takes the decisions and changes the state of the traffic light accordingly. Finally, the Intervention-evaluation use cases apply the Urban Digital Twin to evaluate the effects of certain measures that have been taken to address urban challenges. An example of this would be the use of an Urban Digital Twin to first establish a baseline of air-quality data and then to establish a low-emission zone to reduce air pollution. The Urban Digital Twin can then be used to assess the impact of the intervention.
Decision support horizon
The time interval between the decision horizon and the effect of the horizon is a defining factor to distinguish between various use cases of Urban Digital Twins. We have defined three such horizons: ‘short’ is less than a day, ‘medium’ is between a day and a year and ‘long’ is more than a year. Medium and long-term use-case scenarios have existed in city contexts for a while. The short term horizon, sometimes described as "real-time" (of which the exact time unit depends on what is right for the use case and therefore also called “right-time”) has emerged more recently with the deployment of IoT technologies in urban contexts.
Decision support domain
Various use case domains have emerged as relevant to cities. Air quality was top of mind for many of the consulted stakeholders as the primary application domain for an Urban Digital Twin. Indeed, many cities struggle with problems due to air pollution, caused by e.g., mobility or logistic flows. Not surprisingly, mobility problems like road congestion were also identified as key areas. The city stakeholders also mentioned use cases regarding sound pollution, e.g. the noise produced by students at night around a university. In the water domain, they mentioned use cases related to floods and droughts. Pandemics are also top of mind in cities, as decision support is direly needed to address COVID-19. Tourism was another concern of various cities, aiming to better valorise touristic flows and to guide tourists through the city in specific ways. Finally, city stakeholders also mentioned several use cases related to urban spatial planning, like how to change the city towards paradigms like the “15-minute city”, in which the aim is to allow residents to meet most of their needs within a short walk or bike ride from their place of residence. For completeness, use cases for digital twins can come from any of the Smart City Domains.
End-User types
Various end-users exist that can take decisions pertaining to the city and can therefore be end-user of Urban Digital Twins. First, there are the public servants that work in the various city administrations and that overlook a specific domain of the city. They are specialists in a certain domain, like e.g. mobility or water management. Another type of end-user are policy makers. These are typically people that have been appointed through a political process and of whom the domain knowledge is often less deep than the public servants that provide them with the evidence that is needed to take well-informed decisions. Urban planners, as another distinct end-user type, aim to make long-term, structural changes to the city. Emergency responders are the police, fire department or health services. Citizens represent a final type of end-users of an Urban Digital Twin.
Cross domain decision support as key feature for Urban Digital Twins
Although many of the decision support domains discussed in the previous paragraphs have already been addressed by specific individual information system (IS) in the urban context, these existing IS's usually build on data silos in one particular disciplinary domain. As an example, the city water department and the mobility department often have IS that operate completely independently without any exchange of data. If an exchange of data occurs, this more than often still happens via an asynchronous data extraction, like through csv files that need to be uploaded into the other system. Most of the stakeholders that we consulted recognised that there is value in connecting data sources through a Digital Twin to support decisions. This, we propose, is the main added value of Urban Digital Twins with regards to other pre-existing IS types: they allow interconnecting various urban data sources and modelling algorithms in a way that can grow with the city and reflects its complexity. In doing so, the Urban Digital Twin mirrors the vibrant, complex and evolving nature of its physical urban counterpart. This is all easier said than done, as data exists in a wide diversity of formats, time domains, organisational silos and disciplinary epistemologies. Fortunately, advances in the field of computer science and IS design have provided key design principles on how Urban Digital Twins can be built, on which we will expand later in this paper.
Architecture
In exploring Urban Digital Twins and researching their purpose, we have built a number of prototypes to address end-user needs. A first prototype was an Urban Digital Twin of the city of Antwerp in 2018. It focussed mainly on traffic and air quality. The main idea was that, by changing the traffic situation, this would also affect the air quality. Air quality is a major concern to the city of Antwerp, as a logistic hub through which a big part of Western European freight is transported from maritime ships to trucks and the other way around to and from the hinterland.
A second Urban Digital Twin was built for the city of Pilzen in the Czech Republic. Finally, an Urban Digital Twin is currently being constructed for the city of Bruges, in Flanders. Discussions are ongoing to build Urban Digital Twins for various other cities in Flanders. From the scope analysis with prospective end-users and our experiences with the development of Urban Digital Twins, we have drafted the architecture in Figure 1 as a basis on which to create future Urban Digital Twins.
Data Sources
The proposed Urban Digital Twin architecture has been built with the main objective of supporting cross-domain decision making in mind. In order to do so, it is essential that data sources coming from a number of different domains can be interconnected. This is what is shown in the top part of the architecture, where we see various types of data sources that can be connected in the system. We differentiate between dynamic and static datasources, to indicate that some datasources change a lot and some do not. In the dynamic category, IoT datasources are salient, as they provide the real-time link between the virtual entity (i.e. the city) and its digital representation. In addition to IoT timeseries (a timeseries is a type of dataset in which each sensor measurement is associated with the time at which it was taken), the dynamic data category contains "context data". This is the type of data that is needed to better understand certain sensor measurement, like e.g. the GPS location of where the sensor measurement was taken, the type of sensor or the sensor’s calibration settings. Beside dynamic data, there is static data. In order to build a virtual representation of a city, static data sources like geodata (e.g. 3D model data that represents the structures in a city, like buildings, bridges, etc…) are essential. A lot of the data that pertains to a city resides in other types of static data sources which we have grouped in the category "Urban Data". An example would be demographic data that reflects the number of inhabitants in a certain part of a city as well as the type of inhabitants, categorised according to age, profession, etc.
Although these data sources are currently still siloed in different urban domains, we expect that in the coming years, data spaces with marketplace functionalities will see the light which allow distributed access to datasets. Such data spaces would then handle the details of the value exchange (monetary or open) and would make sure that datasets can be trusted and are offered using common standards. We are already seeing the contours of such developments, for example within the Flemish "Datanutsbedrijf", which translates to “data-oriented utility companies” in English.
Models
With models, we refer to algorithms that add value to the datasets which are part of the Urban Digital Twin and that can be accessed to support cross-domain decisions. Raw data, as produced by e.g. an air quality sensor, is in it itself almost never enough as a basis on which to take decision. Models are necessary to enrich and transform the raw data to support urban decision making. Urban Digital Twins are premised on the idea that they should be able to grow with the city and in time incorporate new models as they are developed. The Urban Digital Twin should thus be extensible in terms of model integration.
Furthermore, models need to be able to use each other's outputs. For example an air quality model that predicts air quality at certain locations at certain times, can greatly benefit from accessing the output of a traffic model that predicts the number and type of vehicles. Being able to interconnect these models and standardise their communication is a crucial part of the Urban Digital Twin research and development roadmap as we perceive it. In terms of model implementations, we distinguish between two types: process-centric and data-centric models.
Process-centric models
These are algorithms, built on human knowledge of real-world processes to make predictions based on data. This can for example be done for air quality, based on an understanding of how crucial parameters like wind, traffic intensity or the 3D layout of a city can influence the air quality at any particular location in the city. The advantage of such process-centric models is that they have been in development and use for decades and that many of them offer accurate results. Their main disadvantage is that they do not have the ability to learn when the real-world environments change, as shown by measured data. Each time a major change in the data emanating from the physical world occurs, the modelled processes need to be re-evaluated and if necessary adapted manually, which can be a long and costly process. Also, the initiative to make changes to the model lies with the human maintainers of the model, who are limited in their ability to grasp real world changes as they are reflected in the large data volumes that are produced by IoT sensor deployments in urban environments. To take into account all the relevant dimensions that are embedded in the data, machines are better suited than humans.
Data-centric models
With the surge in artificial intelligence research and development, data-centric models are becoming increasingly available. Such models learn from the data that is measured through IoT infrastructure that captures the real-time state of the city. As the amount of data that is produced by the IoT networks is often too large for any one person to comprehend, algorithms are necessary that can look at the data as it presents itself and learn from this data the patterns that are needed to improve urban decision making. An example of such an approach is not to build a physical model of air quality, but to measure air quality at a high number of locations in the city and then use an artificial intelligence algorithm to extrapolate to all locations in the city. Such a model knows next to nothing of the processes (wind, traffic, etc...) that influence air quality in an urban environment, but constantly learns patterns based on new incoming data. Data-centric models have the advantage that they can constantly learn from new and changing real-world conditions. Building models that incorporate the human knowledge from process-centric models and at the same time are flexible enough to update this knowledge based on new incoming data is a challenge when creating new Urban Digital Twin models.
Brokers
In order to interconnect various datasets and models, a broker is essential and constitutes the heart of the Urban Digital Twin. A broker is a software component on which data sources can publish data, while consumers of data can subscribe to certain types of data. As the amount of data that is being plugged into the Urban Digital Twin grows, the broker will have more and more data to deal with, which is why performance and scalability are prime concerns when designing and developing brokers. Also, the heterogeneity of dataset types is set to increase over the years, which is why semantics and standards are essential. Brokers are discussed in many different ways in the current Urban Digital Twin domain, which is why we make an attempt at disambiguating the topic in this section.
Context broker
A context broker is an element that was introduced by the FIWare foundation, to manage context data. Context data is a type of “data about data” or meta-data, which allows a better understanding of certain measurements. For example, a raw measurement of air quality is hard to understand, if it does not also include the time and exact location of when the measurement was performed. The main actor in the development of context broker technology in the EU is the FIWare foundation. The FIWare context broker specification has been incorporated in the Connecting Europe Facility initiative, which aims to provide EU countries with reusable building blocks to facilitate the building of digital services. Even though a context broker is important in IoT deployments to contextualise sensors data, the heart of an Urban Digital Twin requires a different type of broker, to which we refer as a data broker.
Data broker
The components within the red circle in the architecture on Figure 1 is what we refer to as a data broker. It does not store data, but collects data from different sources and allows it to be redistributed and understood by various consumers and producers of data. As a data broker is able to handle different types of data sources, a component is needed that allows these data sources to be findable, i.e. a data catalogue. In addition, a data broker needs to be able to make sure that the different data sources are understandable by other components. In order to allow the cross-domain decision making that is the central tenet of the Urban Digital Twin, various datasources and models need to be able to talk to each other. Just like human communication requires semantic alignment to make sure that concepts are understood in the same way between people, so does communication between machines. This is where the knowledge graph comes in, to provide metadata on the different constituents of each datasource. A knowledge graph is constructed of nodes and edges. Each node contains a concept and the edges describe the relationships between concepts. The types of nodes and edges that can be used in a knowledge graph are described in an ontology. An ontology is thus an abstract description of a knowledge graph. At the heart of the data broker is a message broker, also known as a message queue. This is a technology for managing large amounts if incoming data points and redistributing them as needed. Candidates for implementation of the message broker are technologies like Apache Kafka (https://kafka.apache.org/) and systems that apply the OASIS MQTT messaging protocol (https://mqtt.org/software/). Data brokers make datasets findable and accessible but it takes more to unlock the full potential of data, which is why we also need smart data management and governance.
Smart Data management & governance
A digital twin needs smart data management, to make the data interoperable and reusable. Smart data, according to Pieterson (2017) focusses on:
- Utility: the potential utility derived from the data
- Semantics: the semantic understanding of the data
- Data Quality: the quality of the data collected
- Security: the ways data are managed securely
- Data Protection: how privacy and confidentiality are guarded
These are all key aspects to take into account when building Urban Digital Twins. For example, the quality of the data needs to be made explicit. One of the big risks of chaining models is that drift or inaccuracy in one element of the data value chain can lead to error-propagation. This would weaken the ability of an Urban Digital Twin to support accurate decision support. Trust (e.g. data protection) is also a key element to manage and the concept of "verifiable data organizations" is key. To address these concerns, the Urban Digital Twin should use tools and best practices, and align with the state-of-the-art on federated smart data platforms, like the Gaia-X (www.gaia-x.eu) initiative. In order to do so, smart data management and data governance principles should be put in place. Smart data management addresses activities such as schema versioning, data storage at scale (e.g. in data lakehouses), data discovery, publishing, metadata management, querying and data lineage. Data governance is the collection of agreements, processes and rules at the data level on how to maintain and operationally manage the data ecosystem with verifiable data organizations.
Model management & governance
Explicit model management is used to register models, chain their inputs and outputs, train Machine leaning based models in secure sandboxes and to define and register simulation scenarios. These functionalities require model management, service and process management techniques. Workflow and process orchestration are key challenges for the architecture.
Context graph
The context graph allows the different models that exist in the Urban Digital Twin to react to changes that are made by the end-user to the state representation of the city. When the end-user decides to change the properties of the city and want to understand the repercussions, a representation is needed that shows the new and the previous state of the city. Say for example, that the end-user wants to close a street to traffic and understand what this would mean to the air quality in the city: this would require a new traffic layout of the city to be published to the air quality model. The representation of the state of the city is handled by the context graph, while the publication of the changes is handled by the message broker. Microsoft has been one of the most active players in this field, with their Digital Twin Definition Language, which integrates with their Azure Digital Twins offering. Their approach has been to combine the ETSI Saref4City and the ETSI-CIM NGSI-LD ontologies and create a combined DTDL mapping with which context maps can be created.
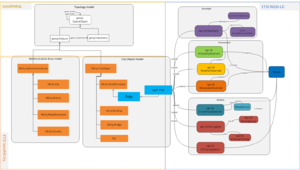
Standards
As the value of an Urban Digital Twin lies in the interconnection of different datasets and algorithms, making sure that their interaction is standardised is key. This is both true at the level of the Application programming interfaces (API) and data models that are used by the various parts of the system as to the data models that are deployed. An API controls the ways in which different components can interact with each other in terms of actions and data. A data model organises data elements to represent properties of real-world entities in an information system, like e.g. how to represent the different parts of a street or a building. Choosing API's and data models that are widely used and supported by a large, relevant and vibrant community is key. There are quite a few components of an Urban Digital Twin and as a result, there are a considerable number of possible standards to look at. We will only discuss the standards that we have been using without aiming to provide an extensive review and comparison of the various relevant standards that are available. An important set of standards comes from the FIWare foundation. This European organisation has proposed the NGSI-LD standard API. It provides a REST API for getting access to IoT data. NGSI-LD provides guidance on how data can be described in terms of form (JSON-LD), while the FIWare data model provide commonly agreed upon ways to describe real-world measurements in terms of content. Many data model have already been described for the cities domain, which provides a relevant set of resources on which to build Urban Digital Twins. [Todo: further expand and discuss the list of used standards]
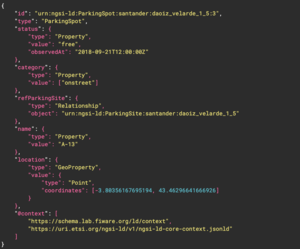
User Interfaces
An often-made assumption is that the main added value of an Urban Digital Twin compared to the state-of-the art in urban IS resides in its user interface and/or the 3D models of the city that it shows. Our position is that this is not the case and that the bulk of the contribution of an Urban Digital Twin resides in the data sharing backend on which the user interface is built. Still, user interfaces for Urban Digital Twins are a challenge. Indeed, the main idea of an Urban Digital Twin is that it constitutes a platform in which gradually multiple datasources and models can be plugged-in to support cross-domain decision making. As we have detailed in the section on end-user types, various types of people are expected to work with the Urban Digital Twin, each with their own set of needs and expectations. As the user interface of the system is the main channel through which such needs and expectations are typically addressed, it means that the Urban Digital Twin user interface must be highly versatile.
Public servants constitute the type of end-user that is most deeply specialised in the domain that they oversee. As a result, they are highly knowledgeable about the type of decisions that they need to take or support and therefore have a capability to wield user interface elements that are powerful, complex and that express concepts that require deep domain knowledge. Beside the public servants, city administrations also count what we have described as policy makers. These are individuals that are typically appointed by some form of democratic process or a derivative thereof and that remain in office for a shorter time than the public servants. Policy makers also want to understand the current state of the city and also need to be involved in decisions that pertain to their city. However, they are less deeply embedded in a specific domain, which means that providing them with deep and complex domain-specific user interfaces may limit their perceived usability of the Urban Digital Twin. Finally, citizens have an interest in understanding what is going on in their city and better understand the impact of decision that have been made. Using an Urban Digital Twin to make decision on the future state of the city is most often beyond the agency of individual citizens. Therefore, they should be provided user interface functionalities that are more about being informed of the impact of future decision, rather than to use the Urban Digital Twin to support decisions of their own. Overall, the different expectations and needs that exist towards the user interface of Urban Digital Twins remains one of the key challenges to address. In addition, the UI needs to be able to deal in a flexible way with the variety of current and future states that the city will reside in. This requires an event publication mechanism that used the semantic data in the context graph to allow the user interface to present the right type of information to each specific type of end-user.
Actuators
Actuators are here to be understood as non-human elements that can effect a change in the real-world. These can be rather “dumb”, like the streetlights in the city, or can have a limited degree of autonomous decision making capability, like an autonomous grocery delivery drone. Actuators require automated (as opposed to human) decision making to function properly. Such decision making can also use the datasets and models that are offered by the Urban Digital Twin. At the time of writing, the number of actuators that are active in cities is very limited. An often-discussed example are traffic lights that react to the state of the traffic that they regulate. However, R&D is actively ongoing at a global scale to introduce new types of actuators in cities, like autonomous vehicles (cars, trucks, barges, trains, trams, drones, …) that transport people and goods. We expect the number of Autonomous actuators that will populate our streets and that will benefit from the presence of an Urban Digital Twin to grow over the coming years.Actuators actuators can have a large positive impact, but can also be a hazard if not functioning correctly. In order to be certain that an automated actuator performs as expected, it can be trained and tested in a virtual simulated environment before it sets out in the real world. This simulation environments is a role which an Urban Digital Twin can play, besides providing researchers with access to clean, standardised and semantically annotated urban datasets.
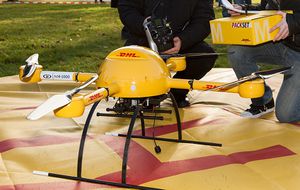
Digital Twin Composition
The definition that we use of a Digital Twin, i.e. as a virtual representation of a physical object, can ultimately apply to many of the physical objects that exist in the real world. This means that over the years, many of the objects that surround us will have a Digital Twin that exists in the digital realm. As a result, Digital Twins can be composable in different ways. By this, we mean that a new Digital Twin can be built that uses the outputs of a number of other Digital Twins. For example, an Urban Digital Twin could build on the outputs of various other Digital Twins of physical objects, like trams, busses, buildings, street lights, etc. Therefore, a main area of investigation for the coming years lies in the interaction of Digital Twins. This is also the idea that is being followed by Cambridge University's Center for the Digital Built Britain (CDBB, https://www.cdbb.cam.ac.uk/DFTG/GeminiPrinciples) and expressed by the FIWare foundation. Following such a Digital Twin composition approach would allow the creation of Digital Twins of an ever greater scale, as is also the objective of the EU's Destination Earth program (https://ec.europa.eu/digital-single-market/en/destination-earth-destine).
Design principles
Through design principles, we mean to discuss various aspects that are worth paying attention to when applying the architectural elements which we have discussed in the previous sections to create Urban Digital Twin instances. A first aspect to take into account is that a once-size-fits-all user interface that suits the needs of all Urban Digital Twin end-user is unlikely to be effective. Instead, user interfaces should be fit for purpose, aiming to strike a balance between power and simplicity that matches the needs of the end user. The various expectations that end users have towards the Urban Digital Twin is also reflected in the responsiveness of the models. Indeed, a trade-off often exists between accuracy of a model and the time it takes to compute. Both process centric and data-centric models can take a long time to compute, even on high-performance computing (HPC) infrastructure. Yet, not all end-user types require the highest degree of accuracy of models. Therefore, when integrating models in an Urban Digital Twin, model responsiveness is an important aspect to consider. To make sure that the lifecycle of the Urban Digital Twin is extended and therefore the investments that are made in its development can yield returns over many years, it is important to make the Urban Digital Twin as open as possible. With this, we mean technical openness, but also organisational openness. The Open Urban Digital Twin should make sure that various components can be integrated over the years, from a variety of organisational actors. This means great emphasis should go to standardising interaction between the various parts of the architecture, but also to make sure that the business models of the actors that contribute to the Open Urban Digital Twin are respected. This means working by default with open data, open source code and open knowledge, but also allowing proprietary contributions where needed. Finally, to allow an evolving set of functionalities of the Open Urban Digital Twin that supports decision making in the city as it evolves over time, the model architecture should be pluggable. By this, we mean that it should be possible to easily integrate new models and to replace operational models with new ones, with minimal effect on the other parts of the Open Urban Digital Twin.
Urban Digital Twin maturity levels
We see currently see 3 Urban Digital Twin maturity levels. Many cities have systems that are at level 1, and quite a few are moving towards level 2. Level 3 remains out of reach for most cities at the moment, yet is expected to become more prevalent in the near future.
Level 1: data dashboard
The first level Urban Digital Twin works with static or dynamic data sources and shows these data sources on a dashboard, using little to no modelling capabilities. This means that the data is presented in the Digital Twin dashboard mainly as it is being measured by the sensors that are deployed in the city.
Level 2: model-based human decision support
In this second maturity level, process-centric or data-centric models are deployed to increase the value of data sources to the decision making process. Different models can be operational in the Urban Digital Twin, provided by various actors. One models can use another model's output and models can be replaced by others without hindering the functioning of the Urban Digital Twin as a whole.
Level 3: model-based human and automated decision support
A final maturity level occurs when the Urban Digital Twins does not only support human decisions, but also allows automated decision support. The Urban Digital Twin is conductive to the training of new algorithms and the testing of their effectiveness, before the algorithms are deployed in the real world. In addition, the algorithms that are plugged in to the Urban Digital Twin can provide the necessary decision support basis to allow the functioning of advanced actuators.
The Urban Digital Twin ecosystem
The discussed architecture can not be delivered by one party alone. Its various constituting parts will be contributed by various actors. We now discuss some of the roles within the Open Urban Digital Twin ecosystem: cloud infrastructure providers, model providers, IoT stack providers, integrators and research institutes. Cloud infrastructure providers are the commercial actors that sell access to cloud services on which the various architectural components can run. Although there is no real reason why cities could not host their Urban Digital Twin "on premises", i.e. on physical servers which they administer themselves, the societal trend has for years clearly been to move to the cloud infrastructure of large US vendors like Amazon, Google and Microsoft. European alternatives like OVHCloud exist, but have less market share than non-EU vendors. Model providers are the companies that specialise in providing access to models in the specific domains that are important to cities (e.g air quality, mobility, sound and water). Many of these model providers currently sell models that come with their own user interface and that do not set out to read outputs from models in other domains or to allow models in other domains to use their outputs. Given a large uptake of Urban Digital Twins that follow specific standards that regulate model interaction, a market could be created in which such model vendors would be able to valorise API access to their models, valorising IP. IoT stack providers are the vendors, developers and maintainers of IoT infrastructure. They specialise in sensor deployments, their maintenance and the quality of their data outputs. Without their continued efforts, there can be no stable reliance on real-time IoT data. Integrators are the development parties that design and develop the various constituting parts of the architecture. They are responsible for making sure the overall Urban Digital Twin functionality is delivered to its end-users. Finally, research institutes are important to drive the innovation in many of the constituting parts of the Urban Digital Twin, as numerous research challenges still exist. A key challenge is in the modelling area. As more real-time sensing data becomes available from various sensors in the city, the possibility arises to create data centric models that constantly learn to improve decision support based on data as it emerges from the city. Machine learning approaches like neural network based supervised learning and reinforcement learning can be instrumental in breaking new modelling ground. Yet numerous other research challenges exist, in both the technological and the organisational context of Urban Digital Twins.
Related initiatives
Besides the above roles, we also would like to point to a number of highly related initiatives of which we are aware.
European Union
At the EU level, the objective of GAIA-X is to create a data sharing infrastructure that would stimulate the European economy and that would be built on European values, e.g. with regard to privacy and trust (von der Leyen, 2020). It aims to work on architectural specifications, development of open source components and certification, all geared towards the inception of trusted data sharing data spaces that allow the development of new applications. Open Urban Digital Twins are a prominent example of the type of application that could be facilitated through GAIA-X, as it builds on the data spaces that are coordinated by it.
Open & Agile Smart Cities (OASC, oascities.org), is a highly relevant initiative, aiming to unite cities beyond country borders to build a global market for data-centric solutions and services. OASC has been working on the development of Minimum Interoperability Mechanisms (MIMs) that are essential to bringing about this market in the cities domain. Context information management (MIM1), Common Data Models (MIM2) and marketplace enablers (MIM3) pave the way for the adoption of Open Digital Twins by creating some of the building blocks that are essential to Urban Digital Twin deployments.
The FIWare foundation has been referred to at various locations in this paper. It aims to encourage the adoption of open standards and open source technologies in various domains, among which cities. The acitivties of FIWare are highly relevant to the development and deployment of Open Urban Digital Twins in cities across the EU and beyond. The EU's Connecting Europe Facility (CEF) aims to boost European digital capabilities by providing a number of reusable digital building blocks that are common to many innovative information systems. CEF has made available a context broker component that can be of use when creating Urban Digital Twins. The context broker CEF building block can certainly be a part of most if not all of the Urban Digital Twin implementations that would be created by various municipalities throughout the EU, like the eID authentication and the EBSI blockchain building blocks.
The European Commission itself will help build cities’ capacity to create their own, “AI-powered local digital twins”, specifically geared towards environmental and climate-related objectives, to help them better understand issues and trends and strengthen the evidence-based analytical capability of policy-makers at local level. In order to build their capacity, preparatory work is ongoing to identify examples from European cities, using Digital Twins for areas relevant to the environment and climate change and to explore how they could be replicated and what combination of generic tools could help in these efforts. In this context, a stakeholder webinar and a technology workshop were organised recently (https://digital-strategy.ec.europa.eu/en/events/workshop-local-digital-twins-technology). At the most recent Digital Day (https://digital-trategy.ec.europa.eu/en/news/eu-countries-commit-leading-green-digital-transformation ), Member States have committed to ….’ work with local authorities and other relevant stakeholders to set up a European network of Digital Twins of the physical environment and support EU cities and regions to use green digital solutions in their transition to climate neutrality’.
Flanders
At the Flemish level, there is the "Datanutsbedrijf" or data utility company which has been commissioned by the Flemish Minister President (Legrand, 2020). The roadmap is currently being drafted and the exact specification are still unclear, but what it already known is that the core intent would be to stimulate the Flemish data economy by allowing more data sharing to occur. This is exactly the type of functionality that is needed for Urban Digital Twins to be able to fulfil their promise of cross-domain decision support. Another initiative that is currently running in Flanders is VLOCA project (https://vloca.vlaanderen.be/), aiming to specify the "Vlaamse Open City Architectuur" together with a wide variety of stakeholders from the quadruple helix. The main objective of this project is to inform Flemish cities on how to avoid situation where their IoT infrastructures would become vendor locked in, without interoperability between stacks and cities, nor data sovereignty. Clearly, Open Urban Digital Twins needs to build on interoperable IoT stacks, which makes the VLOCA initiative highly relevant.
Beyond the European Union
Beyond the EU, Cambridge University's Center for the Built Digital Britain (CDBB) Digital Twin hub, is building up knowledge on how Digital Twins in the built are to be built and interconnected. The focus in this initiative is to connect Digital Twins among each other and to look at standards to do so. At a global level, we note the Digital Twin Consortium (https://www.digitaltwinconsortium.org/), in which different types of Digital Twins are discussed, for example in health, aerospace engineering and infrastructure. The infrastructure work group is particularly relevant to Urban Digital Twins.
Use Cases
Given the above Urban Digital Twin scope, architecture and design principles, we now discuss a number of possible use cases in some of the domains that are top-of-mind in the cities that we have spoken with.
Pandemics
The Luxembourg Institute of Science and Technology (LIST) is leading an initiative regarding the deployment of a Nation-Wide Digital Twin (NWDT) in Luxembourg. The NWDT will serve as both a test-bed and living lab (1) for researchers associated with different research and innovation organizations in Luxembourg, (2) for private and public stakeholders (planners, designers and engineers) testing new products and services, (3) for policy makers using sandboxes to explore different scenarios and to assess the impact of different policies and of new regulatory instruments and (4) for citizens, who have to be fully empowered regarding their participatory governance and the privacy of their data. The COVID-19 pandemic offered an opportunity to illustrate the value of a Nation-Wide Digital Twin in a live real case. A “Cross-Functional COVID Dashboard” has been designed and implemented as a joint effort of Luxembourgish Institutions to give a comprehensive overview of the situation to researchers and stakeholders. This Luxembourgish Nation-Wide Digital Twin offers features (1) to access data, (2) to process data, (3) to visualise data with the goal (4) to support decision making. In the overall concept of the Cross-Functional COVID Dashboard, several verticals such as health, socio-economic impact and logistics have been identified with the domain experts from Luxembourgish Research Institutions and other national stakeholders.
For decision-makers, the purpose was to give the broadest possible overview of an evolving situation that is very difficult to grasp due to its complexity. A specific challenge was to propose the appropriate level of granularity in the data displayed. For researchers, the dashboard helped to assess the quality of the various datasets, to connect the dots between different models and to assess the quality of model outputs to provide the best possible advice to the decision-makers.
A key question to deal with concerned the availability and access to data. Depending on the specific facet of the pandemic under examination, different data sources would be required: (1) existing data produced in the past like socio-economic statistics, (2) data collected via surveys, (3) data produced by simulations based on models. The formats of these data sources proved to be very heterogeneous, and the technical means offered to access them were not all standardised.
A decisive element to the success of this project has been the willingness and the commitment of the stakeholders to give access to the data they owned. The support of the decision-makers of the Research Luxembourg ecosystem (LIST, the Luxembourg Institute of Socio Economic Research LISER, the Luxembourg Institute of Health, LIH, and the University of Luxembourg) and beyond (e.g. Luxinnovation) played a key role in the Luxembourgish context.
A progressive enrichment methodology was followed, starting with a few datasets that were available at the beginning of the crisis (e.g. number of available beds in hospitals) and progressively adding new datasets along the way as they became available. Thanks to the LISER research institute, the impact of the crisis on the global or sectorial GDP was modelled and estimated for several parameters such as the infection rate or the behaviour of cross-border commuters. These models allowed the design of multiple “what-if” scenarios. For instance, what if the cross-border flows are reduced or what if social interactions are restricted? More than 2,000 different scenarios were available for the interactive exploration of the socio-economic consequences of the pandemic.
The Cross-Functional Dashboard is based on a common architecture, which is in turn based on LIST’s DEBORAH middleware to get the data from various sources and to display them on the various interconnected devices available in the LIST Command and Control Room, in particular the wall-sized display system nicknamed viswall (7 m x 2 m, +/- 50 Mio pixels, multi-touch) and interactive tables, as shown in Figure 3. The Cross-Functional dashboard is highly interactive and is particularly suited to support collective decision making in complex situations. The most appropriate visualisation and interaction techniques have been selected to best support the understanding of the ongoing situation as well as to support both researchers and decision-makers in their respective tasks. The latest version of the Cross-Functional Digital Twin Dashboard can display various types of charts illustrating collected data and simulation runs, interactive maps, 3D models of the city, live video streams, and live multimedia streams from social media.
To sum up, the Cross-Functional COVID Urban Digital Twin Dashboard played an important role in raising awareness among national and international stakeholders about the relevance and the need of a Nation-Wide Digital Twin. It has also demonstrated the importance of the collaboration between research institutions and national stakeholders to deliver such a complex, cross-domain initiative.
Air Quality and traffic
A use case which we have encountered in multiple cities in Flanders, is the impact of mobility on air quality. Flanders is a very densely populated area that sees a lot of movement of people and freight. As a result, certain Flemish cities are generally considered to have bad air quality due to car and truck traffic. Low Emission Zones have been installed in multiple urban regions, in which only vehicles that have certain specifications in terms of emissions can circulate. Additionally, circulation plans have been installed to change the way in which traffic moves through the city.
However, there is a lack of decision support tools to understand what the impact of certain changes in urban circulation plans and Low Emission Zones will be on particulate matter or black carbon emission and thus on air quality. Models exist of both how changes in the traffic plan (max speed, traversal direction,...) will influence the amount of vehicles moving through it. Models are also available of how predicted traffic will influence air quality. Still, the traffic prediction models are not linked to the air quality prediction models. Therefore, an important use case in which work is ongoing is to make sure that the output of traffic models can serve as a basis for air quality models in the city of Bruges, in Flanders, Belgium. In 2018, a similar use case has already been deployed as a prototype in the city of Antwerp.
It is important to note that the objective is to obtain a pluggable model architecture, in which an operational traffic model can be replaced by another one, while having minimal effect on the functioning of the air quality prediction model. These models can be provided by research organisations or commercial companies, can be process- or data-centric and can use real-time data or static data.
A concern when integrating air quality models is the fact that they require a lot of computation and can run for hours or days even on High-performance computing (HPC) infrastructure, before results can be observed by end users. The more accurate the model needs to be, the longer the time it takes to compute, which requires a focus on both the fit-for-purposeness of the user interface (not all end-users require the highest accuracy level) and model responsiveness (not all end-users are willing to wait a long time for a response from the model).
In terms of advances in traffic modeling which aligns with the notion of Urban Digital Twin, we refer to the Horizon 2020 Polivisu (https://policyvisuals.eu) and DUET (https://www.digitalurbantwins.com) projects.
Flooding and traffic
As our climate changes, it is predicted that there will be more heavy rainfall during short times in specific areas, causing flash floods. These floods have a large influence on emergency responders, like police, fire department and ambulances. Indeed, floods that occur in streets and roads have a big impact on traffic circulation. Being able to predict such flash floods can be done hours in advance, based on meteorological radar data as well as data that describes the structure of the sewer system and the denivelations in the city, as available from GIS data. The output of this data can then be used to inform the way in which the traffic situation in the city is predicted to evolve in the coming e.g. 3 hours, which is of great value to emergency responders. Both types of models already exist and have been developed by multiple parties, yet the output of the flood prediction models are not connected to the traffic models, which is typically the work that needs to be done in the context of Urban Digital Twins and that will be the focus on the upcoming PRECINCT H2020 project. This project will build on a previous, Flemish funded project called “Flooding” in which the flood prediction models were developed, deployed and tested.
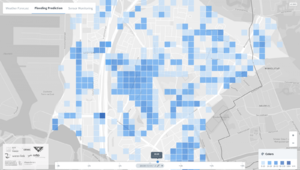
Sound and traffic
Noise pollution generated by traffic is an issue in many cities. This is true for different types of traffic, like cars, trucks, busses and trams. Understanding how changes to the traffic situation will influence the amount of noise pollution at street level can be supported by existing models, yet these are not coupled and can not work with different models from different vendors. Making sure that these models become pluggable in the Urban Digital Twin platform is therefore needed in order to allow them to be integrated in an Open Urban Digital Twin.
Pandemics and people flows
The COVID-19 pandemic has installed the need for cities to monitor and control the number of people that are present at specific locations. This is required to reduce contagion, as the virus will spread more easily in situations where many people are close to each other. Monitoring the location of people can be done by counting them manually, as is currently often done in many cities, to monitor the number of people in shopping streets. However, algorithms are available which can count the number of people in real-time. For example, mobile operator data is available that can indicate the number of people at a city block level. In addition, it is possible to get people counts based on aggregated Wifi MAC address data. Where these technologies could easily be made more accurate and numbers could be offered in a disaggregated and therefore less anonymous way, this would infringe on the privacy of the public and is therefore not an option. Still, data fusion algorithm are becoming available that can take a number of aggregated people flow data sources and refine them into more accurate people counts, while preserving privacy. This allows city decision makers to monitor the flow of people and if necessary take measures, based on a simulation of the result that would ensue after taking certain measures.
Touristic optimalisation
Touristic cities want to understand the spending behaviour of the tourists that visit the city and the provenance of the tourists. Such insights can provide the city with a fine-gained insight in the attractiveness of commercial real-estate in the city to certain tourists groups and the provenance of tourists in general. This can be done by combining cell tower data from mobile operators, with economic demographic provenance data and payment details from payment companies (Haeck, 2021). Both payment and mobile operator cell tower data are highly personal and sensitive and it is therefore absolutely crucial to make sure such use cases are deployed in an Urban Digital Twin with a high respect of citizen privacy, respecting all the legal bounds that apply.
Conclusion
We have aimed to better frame the concept of an Open Urban Digital Twin. We have explained that its main added value is to allow cross-domain decision making and that it can grow with the city to reflect its complexity and vitality. The architecture which we have developed based on ongoing research and implementation projects has been presented and we have explained the various parts of the architecture. We have discussed a number of design principles which can be taken into account when designing and implementing new Urban Digital Twins. Finally, we have have explained a number of Open Urban Digital Twin cross-domain decision support use cases which are important to various consulted cities. Whereas the presented cross-domain use cases are important, they are only examples that scratch the surface of what Open Urban Digital Twins are capable of. A more encompassing approach towards capturing city data and combining these data streams with ML applications will allow for more and more relevant use cases. The question now remains how Open Urban Digital Twins can be deployed in cities across the EU. This will require an investment program which recognises the complex ecosystem that is needed to realise and maintain Open Urban Digital Twins. Achieving this will necessitate, besides the technical challenges, an understanding of the business models that each actor in the Open Urban Digital Twin ecosystem, discussed above, utilise.
References
- Alam, K. M., & El Saddik, A. (2017). C2PS: A digital twin architecture reference model for the cloud-based cyber-physical systems. IEEE access 5, 2050–2062
- Legrand, R. (2020). Vlaams datanutsbedrijf moet uiteindelijk 100 procent privé worden, De Tijd, https://www.tijd.be/tech-media/technologie/vlaams-datanutsbedrijf-moet-uiteindelijk-100-procent-prive-worden/10267823.html
- Grieves, M., & Vickers, J. (2017). Digital twin: Mitigating unpredictable, undesirable emergent behavior in complex systems. Transdisciplinary perspectives on complex systems, 85–113. Springer.
- Haeck, P. (2021), Steden volgen bezoekers tot in hun portemonnee, https://www.tijd.be/ondernemen/technologie/steden-volgen-bezoekers-tot-in-hun-portemonnee/10292453.html
- Pieterson, W. (2017), Being smart with data, using innovative solutions, https://ec.europa.eu/social/BlobServlet?docId=17367&langId=en
- Tao, F., J. Cheng, Q. Qi, M. Zhang, H. Zhang, and F. Sui. (2018). Digital twin-driven product design, manufacturing and service with big data. The International Journal of Advanced Manufacturing Technology, 94(9-12), 3563–3576.
- von der Leyen, U. (2020), State of the Union Address by President von der Leyen at the European Parliament Plenary, https://ec.europa.eu/commission/presscorner/detail/en/SPEECH_20_1655
- Zheng, Y., S. Yang, and H. Cheng. (2019). An application framework of digital twin and its case study. Journal of Ambient Intelligence and Humanized Computing, 10(3), 1141-1153.
- Raes L., Michiels P., Adolphi T., Tampere C., Dalianis T., Mcaleer S., Kogut P. (2021). DUET: A Framework for Building Secure and Trusted Digital Twins of Smart Cities, IEEE Internet Computing
- Lefever S., Michiels P., Van den Berg R. (2019) City of Things: An Open Smart City Vision and Architecture. https://www.imeccityofthings.be/drupal/sites/default/files/inline-files/open_city_vision_paper_final_0.pdf
- Lefever S., Michiels P., Buyle R. (2020) Building Urban Digital Twins. https://inspire.ec.europa.eu/sites/default/files/inspire2020_dt_philippemichiels_rafbuyle.pdf